When your service is completed, you are asked for a rating. Ever thought if your rating can make or mar someone’s career? Your rating is so powerful that it may also change a company’s policy or strategy. Well, that’s the power of ratings which are based on scaling or datascale techniques. These scaling or datascale techniques are so developed so as to ensure that customer level of satisfaction or dissatisfaction is captured. The same is used to take corrective actions or ensure quality services or products are provided to customers to meet their needs.
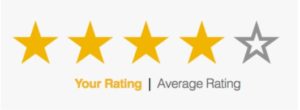
The scaling or datascale techniques are based on the concept of measurement. Measurement can be described as a way of obtaining symbols to represent the properties of persons, objects, events, or states under study. The symbols have the same relevant relationship to each other as the objects under study. These scaling or datascale techniques are used in research methodology to build relevant scales for problem statements.
Example:
Below is how numeric values can define the property understudy or symbols can also define the objects under study. But 3rd table is irrelevant with no relationship establishment.
Number | Property under study |
1 | Male |
2 | Female |
Symbol | Property under study |
M | Male |
F | Female |
The below table is wrong as the symbols do not provide respective uniqueness to the objects under study. Thus, only the above 2 tables are applicable.
Symbol | Property under study |
A | Male |
A | Female |
Table of Contents
What are scaling or datascale techniques?
Scaling or datascale techniques refers to the ability to assign numbers to objects in such a way that:
- Numbers reflect the relationship between the objects with respect to the characteristics involved.
- It allows investigators to make comparisons of the amount and change in the property being measured.
Types of scales
There are 4 primary types of scales – Nominal, Ordinal, Interval, and Ratio. Three important characteristics of the real number system are used to devise the above scales:
- ORDER: numbers are ordered
- DISTANCE: differences between numbers are ordered
- ORIGIN: series has a unique origin indicated by 0 (zero)
- Nominal scale
It is the least restrictive of all scales. It does not possess order, distance, or origin. Numbers assigned serve only as a label or tags for identifying objects, properties, or events.
Example: East -1, West -2, North -3, South – 4
Usage – Permissible mathematical operations: percentage, frequency, mode, contingency coefficients.
- Ordinal scale
It possesses order but not distance or origin. The numbers assigned preserve the order relationship (rank) and the ability to distinguish between elements according to a single attribute & element.
Example:
Companies Ranks
Bata 1st
Shree leather 2nd
Khadims 3rd
Titas 4th
Usage – Permissible mathematical operations: (+) median, percentile, rank correlation, sign test and run test.
- Interval scale
It possesses the characteristic of order and distance but does not possess origin. The numbers are assigned in such a way that they preserve both the order and distance but do not have a unique starting point.
Example: A temperature scale
- 500 F is twice as warm as 250 F
- 100 C is not twice as warm as -3.90 C
Usage – Permissible mathematical operations: (+) Mean, average deviation, standard deviation, correlation.
- Ratio scale
It possesses the characteristic of order distance and origin. The numbers are assigned in such a way that they preserve both the order distance and origin
Example: length (KM scale), weight (KG scale)
- 50 KG is twice as heavy as 25 KG
- 24 pound is twice as heavy as 55.12 pound
Usage – Permissible mathematical operations for all
Types of scaling or datascale techniques
The scaling or datascale techniques are further classified based on Collection techniques and Stimulus.
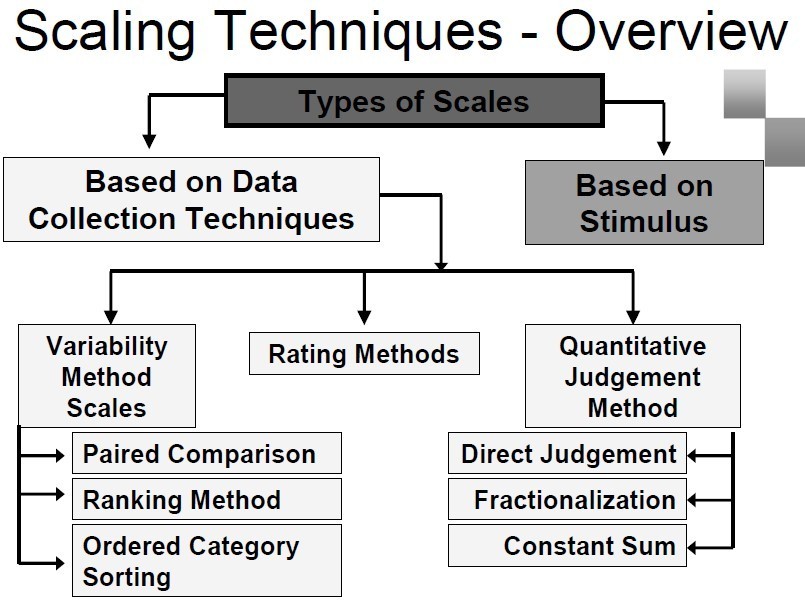
Based on data collection techniques:
Variability Method Scales
It is divided into 3 categories:
- Paired comparison
Respondent to choose one of the pair of stimuli that “dominates” the other with respect to some designated property of interest. Assumptions are that (a)transitivity will be maintained (b) respondent has experience of all the brands on the same attribute
Example: Compare 6 detergent brands on “gentleness on the hands” 6C2 = 15 paired comparisons on the comparison grid.
- Ranking method
It requires respondents to order stimulus with respect to some designated property of study. Assumptions are (a) respondent has experience on all the brands on the same attribute (b) respondents ranking will correctly reflect his preference.
Example: Rank 6 detergent brands on “gentleness on the hands” Normally the respondent is asked to order K/N i.e., Rank top 3 brands (=K) out of the 6 brands (=N).
- Order category sorting
It requires respondents to assign stimulus to ordered categories. It is useful when a large number of stimuli or brands are to be rated.
Example: Assign 6 detergent brands into the following categories – (a) Very
Gentle (b) Moderately Gentle (c) Harsh
Rating Method Scales
It is one of the most popular & easily applied data collection techniques. The respondent is required to place the product or attribute under study on an ordered set of categories and thereby assign a “degree of possessed characteristic” to the attribute under study. Rating scales can be numerical, graphical, verbal, or a mix of all three. It assumes (a) items are being capable of being ranked (b) respondents can psychologically break the ranking into equal intervals (c) scale is ordinal in nature.
Example: The scale is between 0-10, where 10 means that you will definitely buy, 5 you may or may not buy, and 0 means that you will not buy at all.
Very gentle [ ]
Somewhat gentle [ ]
Neither gentle nor harsh [ ]
Slightly harsh [ ]
Very harsh [ ]
Quantitative Judgement Scales
- Direct Judgement scale
It is an advancement on the rating method scale. It assumes that the respondent is able to give a numerical rating with each stimulus with respect to some designated attribute. The scales used are assumed to be interval or ratio scales and it Is normally of two types:
Limited response category – The respondent is limited to choose between one of the given categories.
Unlimited response category – The respondent is free to assume the magnitude of scale and divide it as per his convenience.

- Fractionalisation
The respondent is asked to give numerical estimates to the attributes under study relative to a previously exposed attribute.
Example: Assume that the harshness of brand A is equal to 1. Now rate the relative harshness of the following brands with respect to brand A
B – 1.25, C-0.6, D- 2.50, E-0.90
- Constant sum
The respondent is required to distribute a “number of points usually 100” over a set of alternatives such that the numbers distributed reflect the relative magnitude of importance of alternatives.
Example:
Freshness | 25 |
Cleaning ability | 47 |
Gentle on hands | 12 |
Price | 16 |
Total | 100 |
Based on Stimulus:
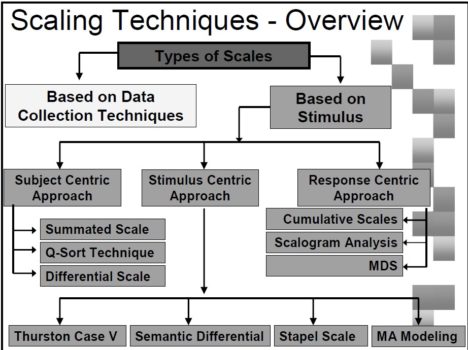
Subject Centric Scales
- Summated scale (Likert scale)
The respondent is required to respond to each of the statements in terms of several degrees of agreement or disagreement. Each response is given a weight that is not disclosed to the respondent. It is similar to the direct judgment method in look and feel and is useful in judging the degree of agreement or disagreement.
Example: To identify the outgoing type of personality please rate yourself on the following statements:
A | B | C | D | E | |
I like playing cricket | 5 | 4 | 3 | 2 | 1 |
I like going to places | 5 | 4 | 3 | 2 | 1 |
I love reading novels | 5 | 4 | 3 | 2 | 1 |
Enjoy life is my motto | 5 | 4 | 3 | 2 | 1 |
I enjoy working alone | 5 | 4 | 3 | 2 | 1 |
Item 1, 2, 4 are favorable and carry (+2 +1 0 -1 -2) as weights.
Item 3 & 5 are unfavorable and carry (-2 -1 0 +1 +2) as weights.
- Sort technique (Stephenson scale)
The respondent is required to sort a set number of statements in predetermined categories (usually 3 / 5 / 7 / 11) – with the restriction that at least a ‘k’ statement should be placed in each
Category. Each category is given a weight and then these weights are used to determine the subject’s attitude towards the attitude under study. Normally used as a precursor to factor/cluster analysis.
- Differential scale (Thurston scale)
It is a modification of the Q-Sort Technique. It assumes that the respondent will agree with a subset of the statements – this agreement in turn reveals the preference of the consumer. The development of the statements for the purpose of the study is done using BLIND Judges.
Most agreed with (Two items) | Neutral (Three items) | Least agreed with (Two items) |
+1 | 0 | -1 |
Stimulus Centric Scales
- Thurston scale – v scaling or datascale model
A scaling or datascale model that allows the construction of a uni-dimensional interval scale from various data collection techniques. It is a fairly complex technique – seldom used. It is based on interval-scaled data. It assumes that “reaction to a stimulus” is normally distributed with a mean (l) and variance (r2). As such we can construct.
RJ – RK = Zjk [Sj 2 + Sk2 – 2 p jk Sj Sk] ^ (0.5)
where RJ, RK = is the response on stimulus J, K
SJ, SK = standard deviation of response J, K
PJK = the correlation coefficient between J and K
ZJK = The normal variate corresponding to J, K
The advantage of using “Thurston Case V” is that it leads to fairly accurate predictions.
- Semantic differential scale
Semantic relates to the study of meaning and the change in meaning. This scale uses “SEMANTIC” to understand the respondent’s “interpretation of meaning”. It allows the researcher to probe both the direction and intensity of respondents’ attitudes using interval scaled data. It is mainly used in image mapping studies.
Example: Understanding the corporate image of BATA
Powerful | x | Weak | ||||||
Modern | x | Old fashioned | ||||||
Warm | x | Cold | ||||||
Reliable | x | Unreliable | ||||||
Careful | x | Careless |
Semantic differential requires extensive pre-testing before it can be put into actual research. Indiscriminate usage may not generate the correct image response leading to the failure of the project.
- Staple scale
It is a modification of the semantic differential scale. It Is an even-numbered non-verbal rating scale used in conjunction with a single adjective. It helps to measure both intensity and direction of response.
Example: How would you rate Bata Stores on “cleanliness”

- Multiattribute modeling
It was proposed by Martin Fishbein in 1967. It uses a mathematical model (usually a linear model) to interpret a person’s attitude on a particular aspect
AO = S BI aI
Where AO is the respondent’s overall attitude towards some object. BI is the respondent’s strength of belief on an attribute. aI is the weight associated with the strength of belief.
Response Centric Scales
- Cumulative scales
It consists of a set of items on which the respondent indicates agreement/disagreement. It is based on the pattern of response – respondent preferences are ascertained.
- Scalogram analysis
It is developed by Louis Guttman in 1958. It is built on the cumulative scale and tries to develop a pattern of “pre-determined responses” by scaling both respondent and responses.
- Multidimensional scaling
It is an advancement over Cumulative and Scalogram Analysis. It tries to determine consumer preferences on more than one dimension simultaneously. It is extremely difficult to develop administer and interpret.
Limitations of Scaling or Datascale Techniques
- Most scales measure attitudes along a single dimension
Human beings are more complex and are normally exposed to more than one stimuli – product features, price, package design, advertising, brand name, etc.
- Scales fail to measure the extraneous influences
Purchase decisions may be made because of pressure from the boss etc. Under such issues – and especially in areas on high involvement goods – scales and measurement may fail completely.
- It is difficult to develop “useable measures” from scales
For example, the question “intention to buy” may not be indicative of market share in the next 6 months. There still exists a divergence between “what scales can capture” and “what market research can deliver”.
FunFact:
The staple scale along with rating method scales are widely used by companies to capture customer reviews.
Let us know what scaling or datascale techniques you come across?